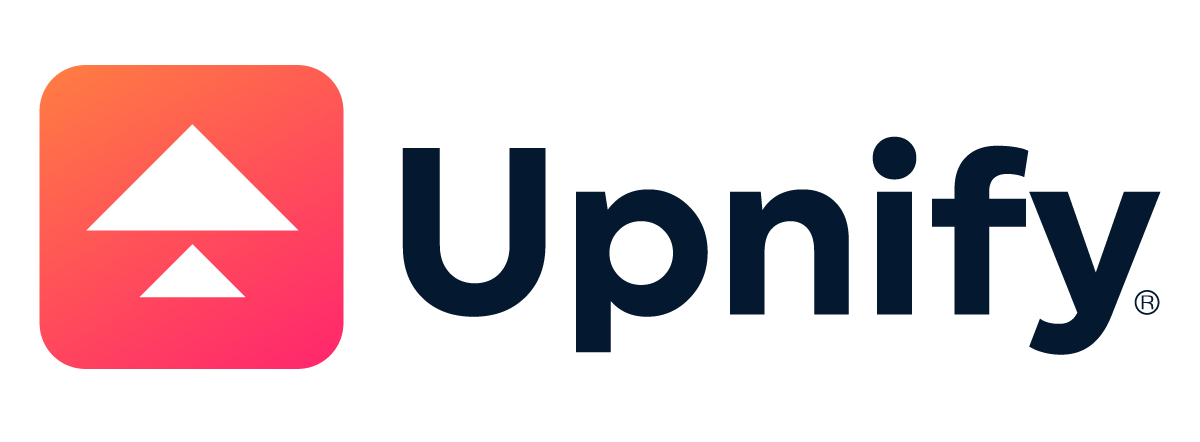
Clustering is a technique used in data analysis and machine learning to identify patterns and structures in unlabeled data sets, also known as clustering. The main objective of clustering is to group objects similar to each other into clusters, so that objects within a cluster are more similar to each other than to objects in other clusters.
The process of clustering involves assigning objects to groups or clusters such that the similarity between objects within a cluster is maximized, while the similarity between objects in different clusters is minimized. The similarity between objects is measured using various techniques, such as Euclidean distance, correlation or cosine similarity, depending on the nature of the data and the clustering algorithm used.
There are different clustering approaches and algorithms, including k-means, hierarchical clustering, DBSCAN, spectral clustering and many others. Each algorithm has its own advantages and limitations, and is selected according to the characteristics of the data and the objectives of the analysis.
Clustering is used in a wide range of applications and fields, such as data mining, customer segmentation, image analysis, bioinformatics, anomaly detection, and data exploration, among others. It provides a way to discover underlying structures and hidden patterns in data, which can be useful for decision making, interest group segmentation and general data understanding.
Importantly, clustering is an unsupervised approach, meaning that it does not require labels or pre-existing information about the data. Instead, it relies on the similarity between objects to form clusters automatically.
The Sales Glossary is a compendium of all the most commonly used terminology in sales strategy. Many of the concepts listed here are used when implementing a CRM system or a digital sales funnel, no matter if they are legacy systems or an online CRM. See also our blog that deals with sales techniques, marketing and sales culture.